The world of kindergarten is abuzz with the magic of free play, where tiny humans learn, grow, and develop essential skills. However, understanding what's really going on during these unstructured play sessions has long been a challenge. Traditional assessment methods often fall short, relying on human observers who might miss the bigger picture. That's where a game-changing new study comes in, leveraging the might of Large Language Models (LLMs) and learning analytics to decode children's self-narratives of their play experiences. This innovative approach analyzed 2,224 play narratives from 29 kindergarten children across four distinct play areas over a semester. The results were nothing short of astonishing: the LLM-based approach achieved an impressive accuracy of over 90% in identifying cognitive, motor, and social abilities. Moreover, the study uncovered significant differences in developmental outcomes across various play settings, highlighting the unique contributions of each area to specific skills. This means that educators can now tap into a wealth of child-centered insights, gaining a deeper understanding of individual developmental trajectories. The implications are profound: by harnessing the power of AI and learning analytics, teachers can create personalized learning plans that cater to each child's unique needs, revolutionizing early childhood education. Imagine a future where every child thrives, empowered by a tailored educational experience that unlocks their full potential. This pioneering study is just the beginning, paving the way for a brighter, more adaptive, and more effective approach to kindergarten learning.
CYBERNOISE
Validating the Effectiveness of a Large Language Model-based Approach for Identifying Children's Development across Various Free Play Settings in Kindergarten
Imagine a world where AI helps teachers tailor education to each child's unique needs - and it's already happening! A pioneering study is transforming kindergarten learning by harnessing the power of Large Language Models to decode the secrets of playtime.
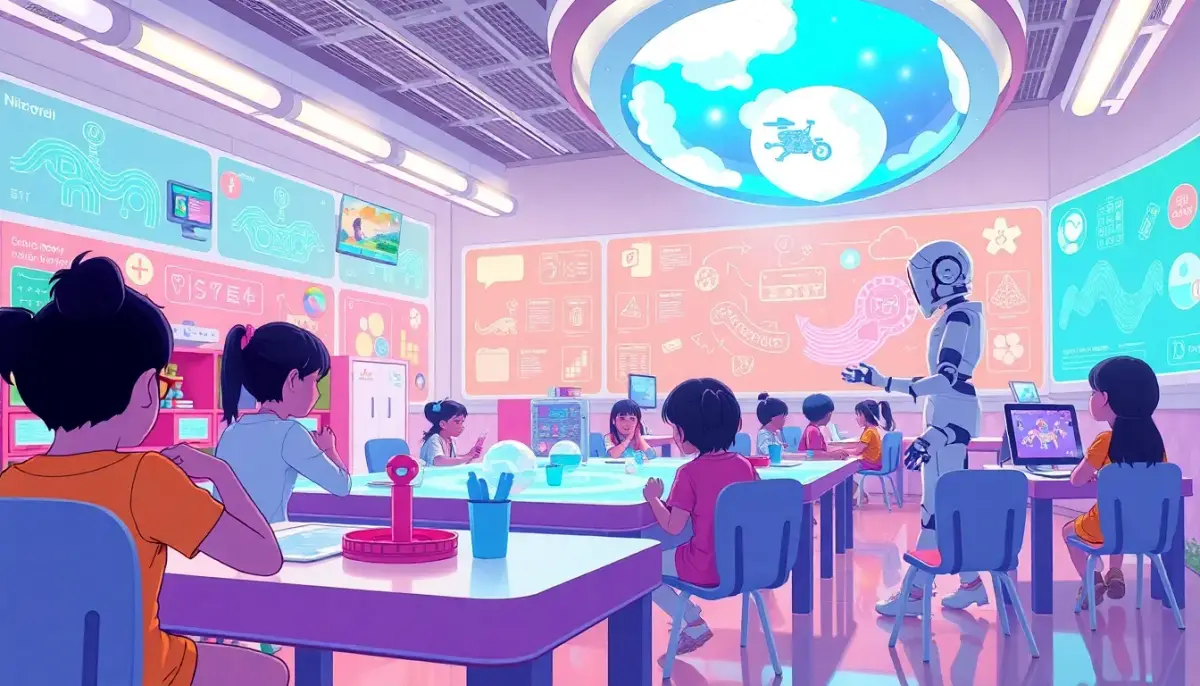
Original paper: https://arxiv.org/abs/2505.03369
Authors: Yuanyuan Yang, Yuan Shen, Tianchen Sun, Yangbin Xie