The rise of AI-powered mental health detection has been a game-changer in the medical field, offering a non-invasive and cost-effective way to assess mental well-being. However, these models have been plagued by a major flaw: gender bias. This has led to inaccurate predictions and unfair treatment, which can have serious consequences. But what if we told you that researchers have cracked the code to mitigating this bias? By leveraging domain adversarial training, a cutting-edge technique that treats different genders as distinct domains, scientists have created an AI model that's more accurate and fair than ever before. The results are staggering, with the new model boasting an F1-score that's 13.29 percentage points higher than the baseline. This breakthrough has far-reaching implications, paving the way for more effective mental health assessments and treatment plans. Imagine being able to detect mental health issues earlier and more accurately, allowing for timely interventions and better patient outcomes. The potential is vast, and we're excited to see where this technology takes us. With the ability to integrate this model into existing healthcare systems, we're on the cusp of a revolution in mental health care. The possibilities are endless, and we're honored to be a part of this journey. As we move forward, it's clear that AI will play an increasingly important role in shaping the future of mental health detection and treatment. And with this new development, we're one step closer to creating a more compassionate and effective healthcare system. By harnessing the power of AI, we can create a brighter, more inclusive future for mental health care.
CYBERNOISE
Domain Adversarial Training for Mitigating Gender Bias in Speech-based Mental Health Detection
Imagine a future where AI helps doctors detect mental health issues like depression and PTSD with unprecedented accuracy, regardless of your gender. Sounds like science fiction, right? Well, that future is now! Thanks to a pioneering new approach, we're one step closer to revolutionizing mental health assessments.
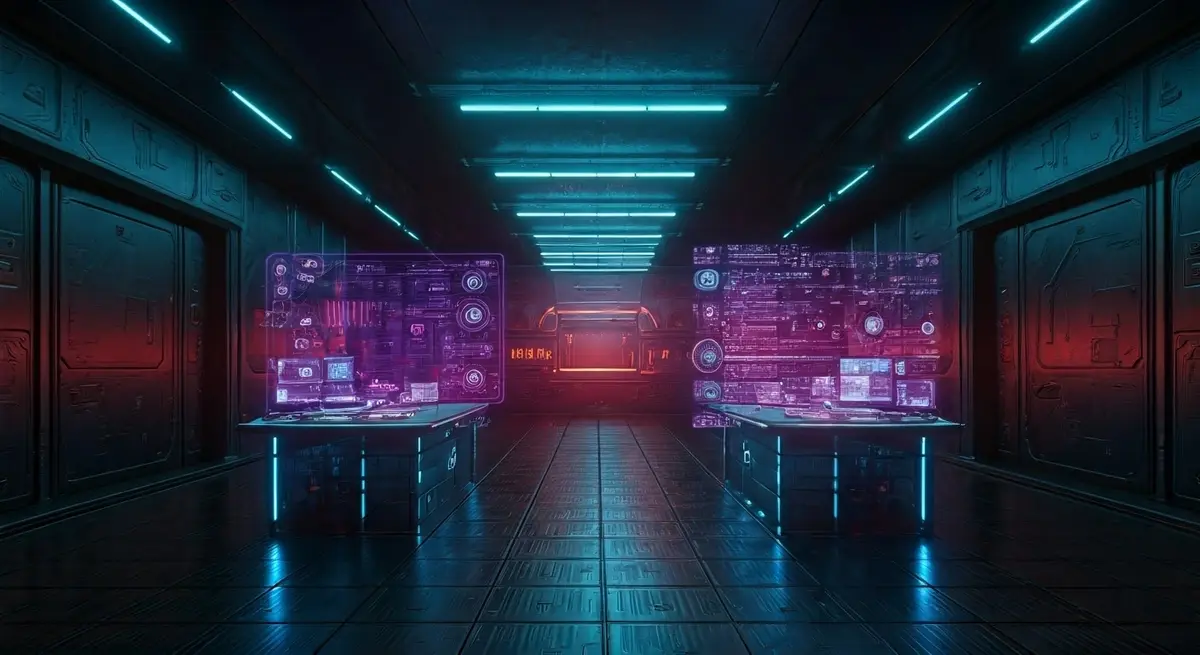
Original paper: https://arxiv.org/abs/2505.03359
Authors: June-Woo Kim, Haram Yoon, Wonkyo Oh, Dawoon Jung, Sung-Hoon Yoon, Dae-Jin Kim, Dong-Ho Lee, Sang-Yeol Lee, Chan-Mo Yang