In the ever-evolving landscape of artificial intelligence, the ability to control and fine-tune Large Language Models (LLMs) has become a holy grail for researchers and developers. The complexity and opacity of these models have long presented a significant challenge, making techniques like fine-tuning both resource-intensive and cumbersome. However, a groundbreaking approach has emerged in the form of Contrastive Activation Engineering (CAE), promising to revolutionize the way we interact with and steer LLMs. By applying targeted modifications to the internal representations of these models at inference time, CAE offers a flexible, task-specific tuning capability without the hefty computational price tag. But how effective is this technique, and what are its limitations? Recent studies have shed light on the performance of CAE in both in-distribution and out-of-distribution settings, highlighting its potential while also cautioning against its drawbacks. The findings are nothing short of fascinating: CAE shines brightest when applied within familiar contexts, with its effectiveness plateauing after a certain number of samples are used to generate steering vectors. However, it's not without its vulnerabilities, including susceptibility to adversarial inputs and a negative impact on model perplexity. Moreover, larger models exhibit a greater resilience to the degradation induced by steering. As we stand on the cusp of this new frontier in AI tuning, the guidelines for effective CAE deployment are beginning to take shape. By understanding the patterns and mechanisms that underpin CAE, we can unlock a future where AI models are not just powerful, but also agile and adaptable to our needs. The implications are vast, from enhancing the performance of AI in specific tasks to mitigating the risks associated with model opacity. As we move forward, the promise of CAE beckons: a future where the full potential of LLMs can be realized with unprecedented precision and flexibility.
CYBERNOISE
Patterns and Mechanisms of Contrastive Activation Engineering
Imagine having the power to steer the behavior of AI models with a mere tweak, unlocking new possibilities for task-specific performance without breaking the bank on computational resources. The future of AI is here, and it's all about mastering Contrastive Activation Engineering.
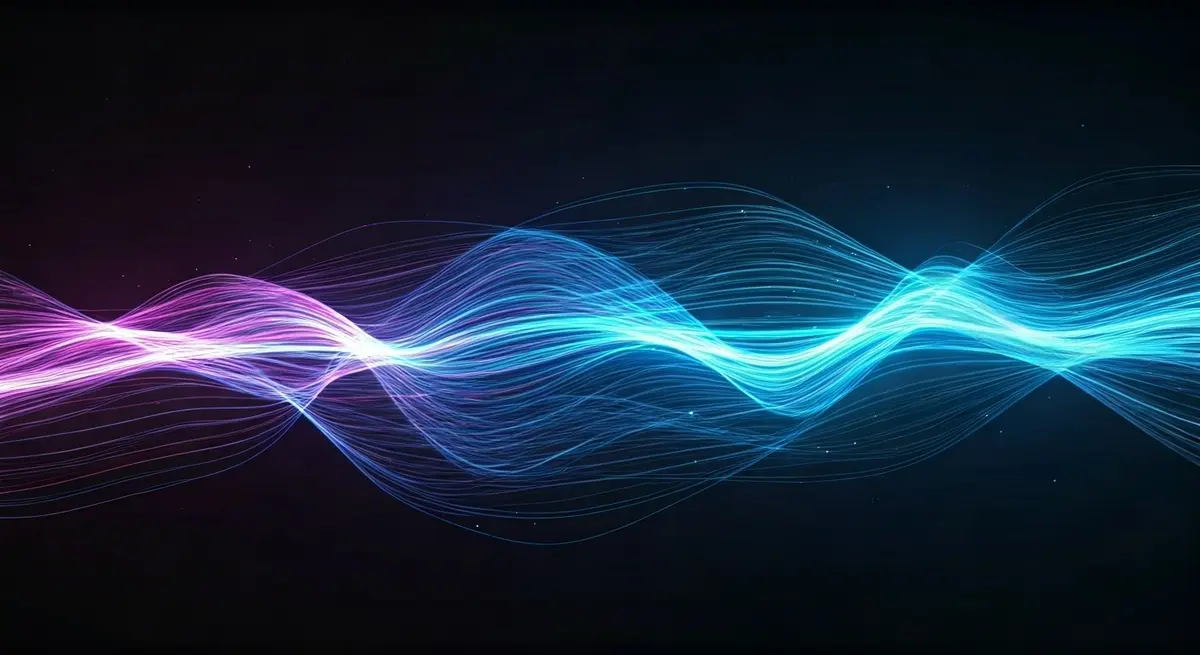
Original paper: https://arxiv.org/abs/2505.03189
Authors: Yixiong Hao, Ayush Panda, Stepan Shabalin, Sheikh Abdur Raheem Ali