The world of mathematics is on the cusp of a revolution, and it's all thanks to the rise of neurosymbolic AI. By combining the power of large language models with formal reasoning, researchers have achieved human-level performance on math competition problems in algebra, geometry, and number theory. But there's a new challenge on the horizon: combinatorics. To tackle this, the CombiBench benchmark has been introduced, comprising 100 combinatorial problems formalized in Lean 4 and paired with their corresponding informal statements. This comprehensive benchmark covers a wide range of difficulty levels, from middle school to university level, and spans over ten combinatorial topics. It's the perfect testing ground for AI's IMO solving capabilities, featuring all IMO combinatorial problems since 2000 (except IMO2004 P3). With the Fine-Eval evaluation framework, researchers can assess AI's performance on both proof-based problems and fill-in-the-blank questions. The results are promising, with Kimina-Prover achieving the best results among tested models, solving 7 problems out of 100. Although there's still a long way to go, this breakthrough paves the way for a new era in AI-driven mathematics. As researchers continue to push the boundaries, we may soon see AI tackling the most complex combinatorial problems, unlocking new insights and discoveries. The future of math has never looked brighter!
CYBERNOISE
CombiBench: Benchmarking LLM Capability for Combinatorial Mathematics
Imagine an AI that can solve the most baffling combinatorial math problems, unlocking new secrets of the universe! Sounds like science fiction, but it's becoming a reality. Dive in to discover the latest breakthrough!
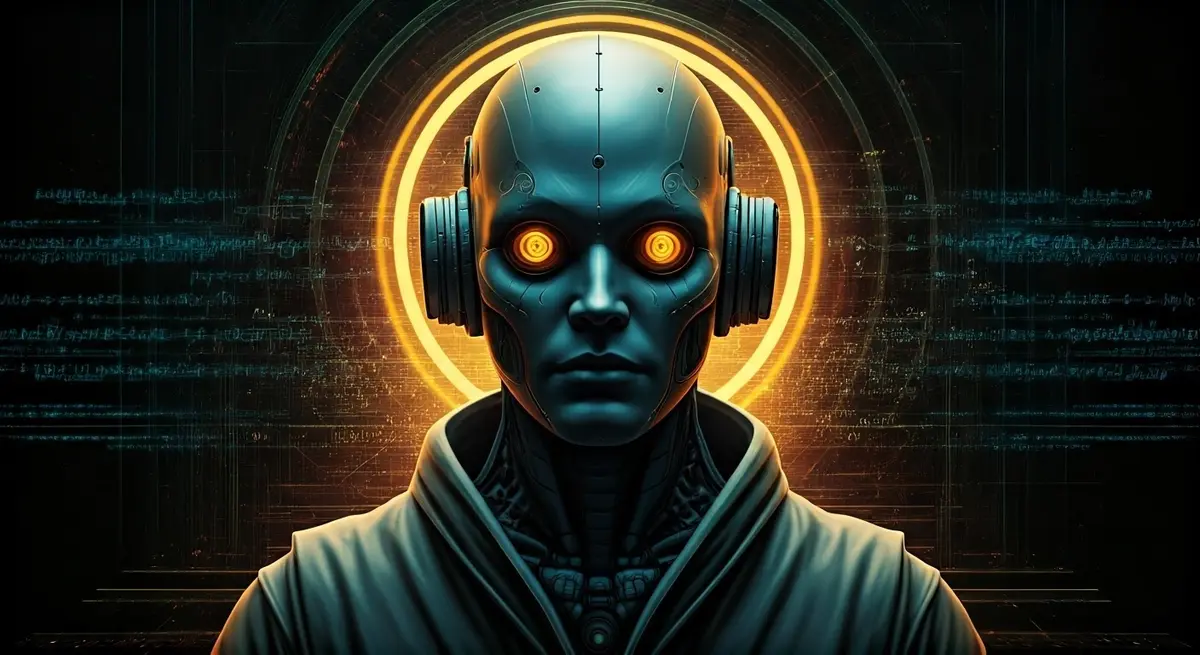
Original paper: https://arxiv.org/abs/2505.03171
Authors: Junqi Liu, Xiaohan Lin, Jonas Bayer, Yael Dillies, Weijie Jiang, Xiaodan Liang, Roman Soletskyi, Haiming Wang, Yunzhou Xie, Beibei Xiong, Zhengfeng Yang, Jujian Zhang, Lihong Zhi, Jia Li, Zhengying Liu