In a major breakthrough, researchers have developed a cutting-edge framework that leverages mixed integer optimization (MIO) to revolutionize the detection and estimation of structural breaks in time series regression models. This innovative method is poised to transform the field of data analysis, enabling professionals to uncover hidden patterns and trends with unparalleled precision. By reframing the $l0$-penalized regression problem as a quadratic programming problem with integer- and real-valued arguments, the MIO framework can identify provably optimal solutions using a well-established optimization solver. Unlike traditional methods, such as $l1$-penalized regression (LASSO), this novel approach allows for the simultaneous estimation of the number and location of structural breaks, as well as regression coefficients, all while accommodating user-specified or minimal number of breaks. The asymptotic properties of the estimator have been rigorously derived, and extensive numerical experiments have demonstrated its superiority over popular non-MIO alternatives, showcasing a more accurate estimation of multiple breaks. To illustrate its practical applications, two empirical examples are presented, highlighting the framework's utility in business and economic statistics. As data-driven decision-making continues to gain prominence, this pioneering method is set to empower professionals across industries to extract valuable insights from complex data, driving innovation and growth. With its vast potential, this breakthrough is expected to have far-reaching implications, transforming the way we analyze and interpret time series data. As we look to the future, the possibilities are endless, and the impact of this technology is sure to be felt across various sectors, from finance to healthcare, and beyond.
CYBERNOISE
Change-Point Detection in Time Series Using Mixed Integer Programming
Imagine having the power to pinpoint exact moments when trends shift and patterns change in complex data. Get ready to unlock the future of data analysis with our game-changing approach!
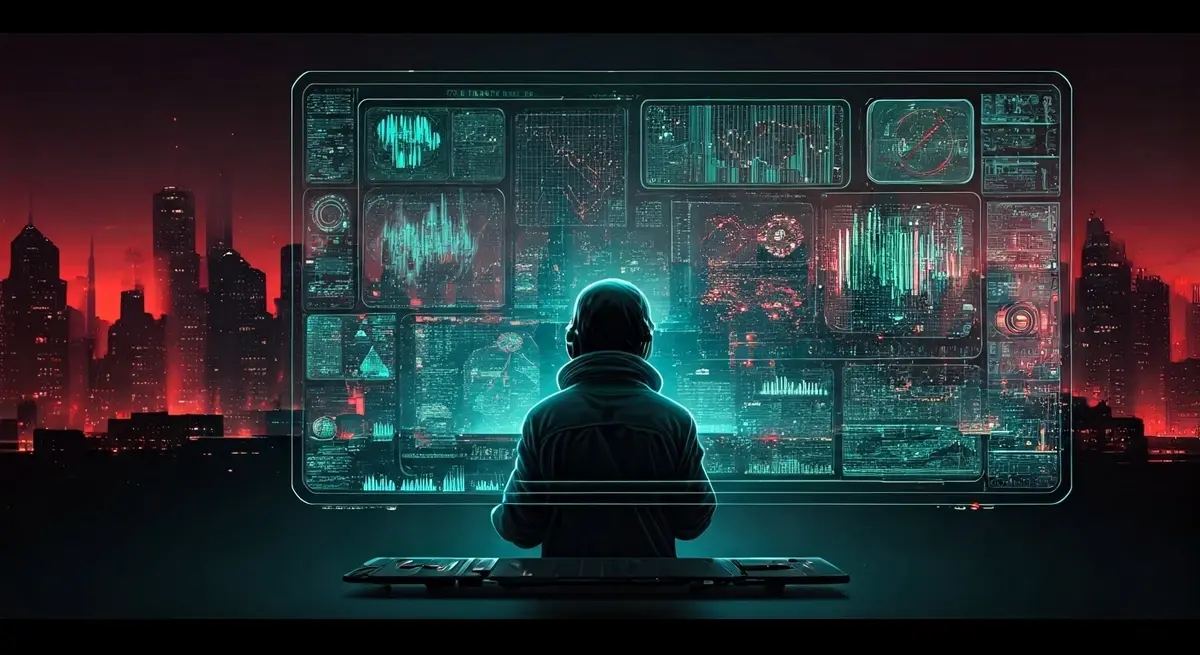
Original paper: https://arxiv.org/abs/2408.05665
Authors: Artem Prokhorov, Peter Radchenko, Alexander Semenov, Anton Skrobotov