In a leap forward for artificial intelligence, researchers have harnessed the power of graph neural networks (GNNs) to crack the code of causal inference in complex networks. The implications are staggering, from optimizing urban planning and epidemiology to streamlining financial transactions and predicting social dynamics. Until now, understanding cause and effect in vast networks was a daunting task due to the confounding variables that muddy the waters. The breakthrough comes from recognizing that interference between nodes in a network decays with distance, allowing for the application of shallow GNN architectures that can tease out the underlying structure. This isn't just a minor tweak; it's a fundamental shift in how we approach problems of causality. By leveraging GNNs, scientists can now adjust for the confounding effects of network connections and covariates across all units, a high-dimensional problem that was previously thought to be intractable. The beauty of this approach lies in its simplification of a complex problem into a manageable form, using the network's own structure to reveal the hidden patterns. Think of it as using the network's blueprint to unravel the tangled threads of causality. The optimism surrounding this development is palpable, as it opens the door to more accurate forecasting and decision-making across various domains. With the ability to dissect complex systems with precision, we edge closer to a future where data-driven insights become the norm. The study represents a confluence of advancements in AI, network science, and statistical analysis, demonstrating the potential for interdisciplinary research to yield transformative results. As we stand on the cusp of this new frontier, the possibilities seem endless. From enhancing our understanding of social networks to optimizing infrastructure, the applications of this technology are poised to reshape our world in meaningful ways. The future isn't just about bigger data or more complex models; it's about leveraging the right tools to uncover the insights that will propel us forward.
CYBERNOISE
Graph Neural Networks for Causal Inference Under Network Confounding
Imagine being able to predict the ripple effects of a single action across a vast network of interconnected nodes. The future of causal inference has arrived, and it's powered by AI. Get ready to uncover the hidden patterns that shape our world.
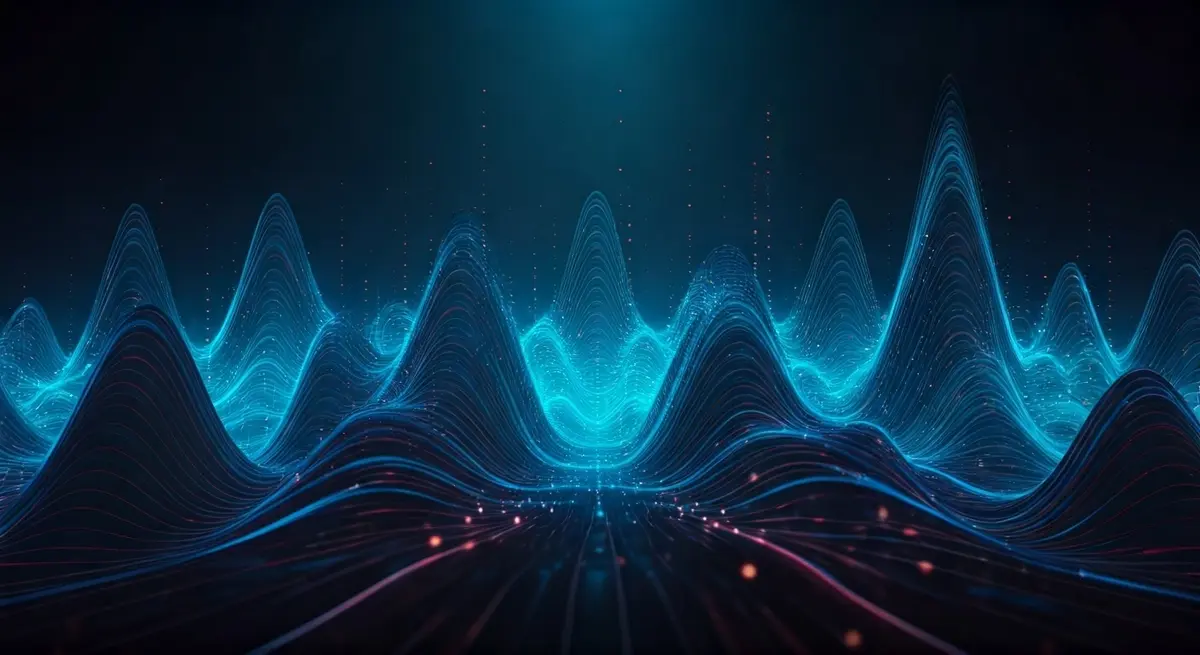
Original paper: https://arxiv.org/abs/2211.07823
Authors: Michael P. Leung, Pantelis Loupos